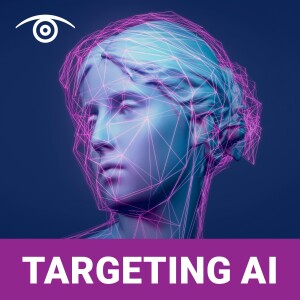
Monday Mar 25, 2024
Security, bias risks are inherent in GenAI black box models
From bias to hallucinations, it is apparent that generative AI models are far from perfect and present risks.
Most recently, tech giants -- notably Google -- have run into trouble after their models made egregious mistakes that reflect the inherent problem with the data sets upon which large language models (LLMs) are based.
Microsoft faced criticism when its models from partner OpenAI generated disturbing images of monsters and women.
The problem is due to the architecture of the LLMs, according to Gary McGraw, co-founder of the Berryville Institute of Machine Learning.
Because most foundation models are a black box that contain security flaws within their architecture, users have little ability to manage the risks, McGraw said on the Targeting AI podcast from TechTarget Editorial.
In January, the Berryville Institute published a report highlighting some risks associated with LLMs, including data debt, prompt manipulation and recursive pollution.
"These are some risks that need to be thought about while you're building your LLM application so that you don't put your business, your enterprise, your business, at more risk than you want to take on when you adopt this technology," McGraw said.
The risks are embedded in both closed and open source models and small and large language models, he added.
"When people build their own language model, what they're often doing ... is taking a foundation model that's already developed and they're training it a little bit further with their own proprietary prompting," he continued. "These steps do not eradicate the risks that are built into the black box. In fact, all they do is hide them even further."
These risks can be dangerous for real-world situations such as the 2024 election, McGraw said. Since the language models are built from data from all over the web -- both good and unreliable -- LLMs trained on that data can be used to produce false and malicious information about the election.
"Using this technology, we need some way of controlling the output so that it doesn't get back out there into the world and just cause more confusion among people who don't know which way is up," he said.
Esther Ajao is a TechTarget Editorial news writer and podcast host covering artificial intelligence software and systems. Shaun Sutner is senior news director for TechTarget Editorial's information management team, driving coverage of artificial intelligence, unified communications, analytics and data management technologies. Together, they host the Targeting AI podcast series.